How to Effectively Use AI for Predictive Lead Scoring: A Comprehensive Guide for B2B and SaaS Startups
Executive Summary: In the increasingly competitive landscape of B2B and SaaS markets, efficient lead management is paramount for startups striving for sustainable growth. Artificial intelligence (AI) is rapidly transforming various aspects of business operations, and its application in predictive lead scoring presents a significant opportunity to optimize sales processes. This comprehensive guide details the evolution of predictive lead scoring, its advantages over traditional methods, critical data types, AI algorithms, implementation steps, key performance indicators (KPIs), challenges, best practices, and real-world examples of successful adoption. By embracing AI-driven lead scoring, startups can enhance sales efficiency, improve conversion rates, and reduce customer acquisition costs.
The Evolution and Advantages of Predictive Lead Scoring
Predictive lead scoring is a modern approach to lead management that employs AI and historical data to identify and prioritize leads with the highest potential for conversion. Unlike traditional scoring—which relies on a fixed set of manually defined criteria—predictive models continuously learn from actual outcomes and update their predictions in real time. This dynamic process enables a more objective and accurate evaluation by analyzing a wide range of behavioral, demographic, and firmographic signals.
Defining and Contrasting Predictive Lead Scoring
Traditional lead scoring models are often based on human assumptions and static rules. In contrast, predictive lead scoring harnesses machine learning to detect complex patterns in historical data, eliminating much of the bias inherent in manual scoring. For further insights on how AI transforms these processes, check out this detailed piece on AI lead scoring from Demandbase.
Key Benefits for B2B and SaaS Startups
Implementing AI for predictive lead scoring offers significant benefits. Sales teams can focus on leads with a demonstrably higher propensity to convert, thereby improving conversion rates and shortening the sales cycle. Moreover, aligning marketing and sales teams around a unified, data-driven lead evaluation process ensures better resource allocation and reduced customer acquisition costs. For a deeper dive into these benefits, visit EngageBay.
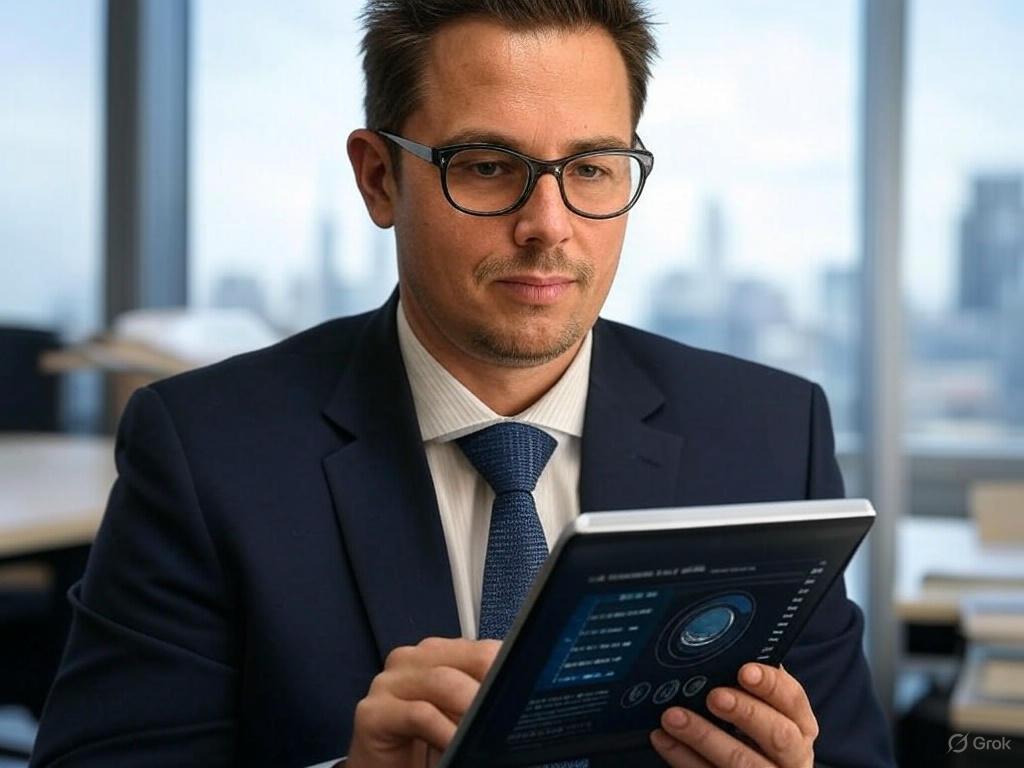
Unlocking Precision: How AI Powers Predictive Lead Scoring
AI and machine learning lie at the core of predictive lead scoring, enabling automated data analysis and the discovery of intricate patterns. These algorithms continuously improve their accuracy by learning from new data, which provides a level of objectivity and real-time adaptability not found in traditional models. This automated approach frees teams from manual recalibration and allows constant optimization as market and customer behaviors evolve.
Crucial Data Types for AI-Driven Predictions
- Demographic DataInformation about the lead such as age, job title, and location.
- Firmographic DataCompany size, industry, and revenue metrics to assess organizational fit.
- Behavioral DataEngagement signals like website visits, content downloads, and email interactions.
- Engagement & Intent DataData indicating active interest such as webinar attendance or demo requests.
- Technographic and Psychographic DataTechnology usage and underlying attitudes or preferences that influence buying decisions.
Navigating the Algorithms: AI Techniques for Effective Lead Scoring
Logistic Regression
Logistic regression provides a balance between interpretability and predictive power. It estimates the probability of conversion based on input features, making it a great starting point for many startups. However, its linear assumptions may not capture all the intricate relationships within the data.
Decision Trees and Random Forests
Decision trees offer a clear visual approach by breaking down the decision-making process into understandable steps. Random forests, an ensemble of decision trees, improve predictive accuracy and reduce overfitting. These methods are effective for identifying non-linear patterns but may sacrifice some interpretability.
Neural Networks and Deep Learning
Advanced neural networks, including Graph Neural Networks (GNNs), can uncover highly complex, non-linear relationships in lead data. Although they demand more computational power and expertise, their ability to process vast datasets in real time makes them particularly powerful for predictive lead scoring. For an industry perspective on the shift to AI-powered approaches, read this article on Forbes.
From Theory to Practice: Implementing AI Predictive Lead Scoring in Your Startup
Step-by-Step Guide
1. Define clear objectives such as improving conversion rates or shortening the sales cycle. 2. Integrate data sources from CRM systems, marketing platforms, and web analytics. 3. Choose the appropriate AI algorithm based on your data characteristics. 4. Train the model with historical data from both converted and non-converted leads. 5. Deploy the model within your CRM to enable real-time scoring and continuous refinement. For detailed technical guidance on model configuration and deployment, refer to the resources available on Microsoft Learn.
Measuring Success and Overcoming Challenges
Key Performance Indicators (KPIs)
- Lead Conversion RateMeasures the percentage of leads that convert into paying customers.
- Sales Cycle LengthTracks the time from initial contact to deal closure.
- Marketing ROIAssesses the revenue generated relative to the marketing investment.
- Win Rate and Lead-to-Opportunity RatioAdditional metrics to fine-tune the model’s predictive accuracy.
Challenges and Best Practices
One of the primary challenges is ensuring data quality and completeness. Inaccurate or sparse data can lead to flawed predictions. Regular data cleaning, validation, and enrichment are essential. Moreover, balancing model complexity with interpretability is crucial to maintain trust among sales and marketing teams. Continuous monitoring and iterative retraining of the model help address evolving market conditions and customer behaviors.
By following these best practices and leveraging the transformative power of AI, B2B and SaaS startups can revolutionize their lead management processes, driving revenue growth and operational efficiency.
In conclusion, predictive lead scoring powered by AI offers a dynamic, accurate, and efficient method for prioritizing high-value leads. Embracing this approach enables startups to optimize their resource allocation and ensure that both marketing and sales teams focus on opportunities that are most likely to convert.